
Queen's Tower, summer 2022. By B Li.
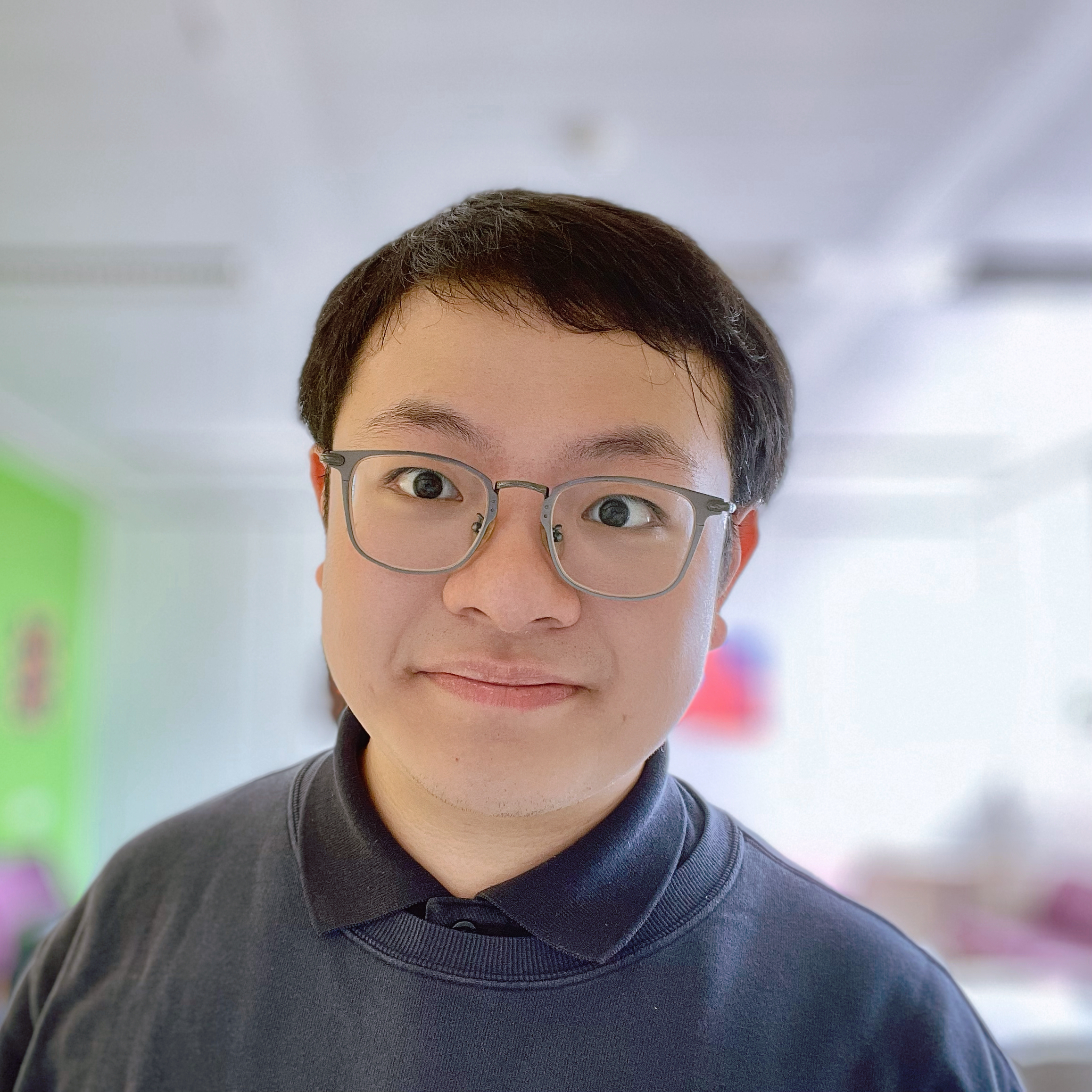
Binghuan Webster Li [李 秉桓]
binghuan.li19 [at] imperial.ac.uk
I am a Research Postgraduate (Ph.D.) and a Chemical Engineering Scholar @ The Xu Group (Bio-Fluid and Transport), Department of Chemical Engineering, Imperial College London.
I received my MEng in Biomedical Engineering (First Class Hons.) from the Department of Bioengineering (2019-23), with two years of research experience @ Cardiovascular Biomechanics and Ultrasound Laboratory (2021-23). The title of my MEng thesis is Surrogate Modelling of Fluid Dynamics within Various Vascular Geometries with Physics-Informed Neural Networks (June, 2023), supervised by Dr Choon Hwai Yap. The abstract can be found here.
My current research features the deployment and integration of computational mechanics frameworks to study the haemodynamics and mechanical responses associated with the aortic-related pathologies. Prior to my current position, I worked on the modelling of flow dynamics within human cardiovascular structures, through the realisation of multiscale computational approaches, including computational fluid dynamics and physics-informed machine learning.
I received my MEng in Biomedical Engineering (First Class Hons.) from the Department of Bioengineering (2019-23), with two years of research experience @ Cardiovascular Biomechanics and Ultrasound Laboratory (2021-23). The title of my MEng thesis is Surrogate Modelling of Fluid Dynamics within Various Vascular Geometries with Physics-Informed Neural Networks (June, 2023), supervised by Dr Choon Hwai Yap. The abstract can be found here.
My current research features the deployment and integration of computational mechanics frameworks to study the haemodynamics and mechanical responses associated with the aortic-related pathologies. Prior to my current position, I worked on the modelling of flow dynamics within human cardiovascular structures, through the realisation of multiscale computational approaches, including computational fluid dynamics and physics-informed machine learning.